Can We Detect Fraud in the Blockchain Using Machine Learning? | by Noah Mukhtar | Jan, 2023
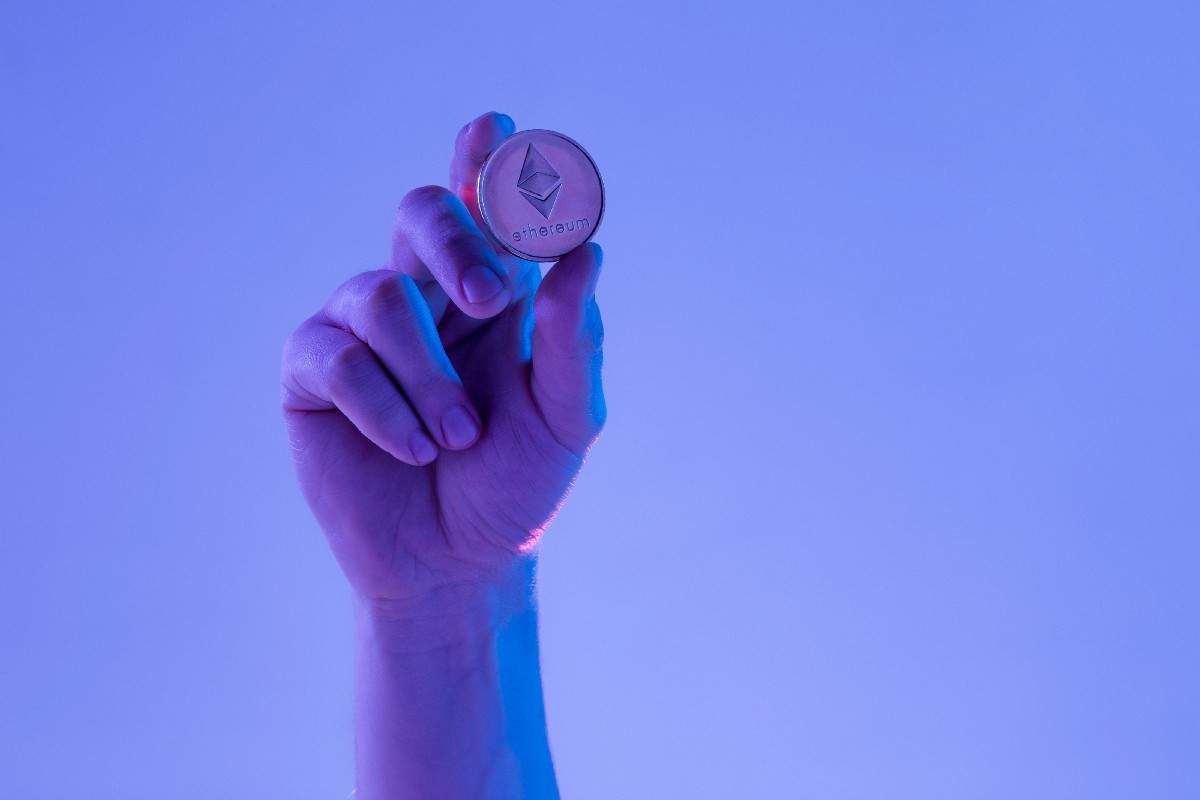
#Detect #Fraud #Blockchain #Machine #Learning #Noah #Mukhtar #Jan
An Elaborate Guide on How To Catch Fraudsters Using an Ethereum Dataset & Machine Learning
Since the emergence of blockchain, it has never been more seamless for companies, banks, and customers to trade goods and transfer money. With this new era of e-commerce, the blockchain has acted as an attractive alternative that bypasses traditional intermediaries, and with that, we discover new ways to commit financial crimes, and with the vast collection of data we have today, we need to develop new ways to beat them.
Is Fraud Changing?
Fraudsters are constantly on the hunt for new mediums to commit crimes, and with the arrival of the blockchain they have managed to find a new way to exploit its potential for laundering money & committing fraud.
Bad actors are concealing their trail through one of the community’s most highly accredited tokens: Ethereum.
Can Ethereum Be Exploited?
Ethereum’s blockchain technology has rapidly exploded in popularity over the past two years despite having protocols that are “uniquely vulnerable to hacking” due to their open source code, large pools of assets, and rapid growth that may have lead to a lapse in security best practices.
Is There a Rise in Crime?
A staggering $1.9b worth of cryptocurrency was stolen in the first seven months of 2022, 60% higher than the same period in the year prior.
“Decentralized finance” (DeFi) protocols (i.e., including Ethereum) were accountable for 17% of all funds sent from illicit wallets, and the quick swapping nature between different types of cryptocurrencies only lended itself useful for launderers.
Why Do We Need Data Science?
It is imperative to find hidden patterns in data to prevent fraudulent transactions from happening in the first place. This might be as simple as detecting unusual transaction patterns relevant to usual spending behaviours, or as complex as detecting when a hacker attempting to modify a process block in the blockchain (i.e., tampering a transaction and its corresponding hashes on the blockchain)
The following steps explain the approach in data construction:
Our dataset is sourced from Ethereum Blockchain records and contain 9,841 rows, of which only 7,662 (i.e., ~80%) are legitimate.
Problem: Imbalanced Dataset
Our dataset is highly imbalanced, making the model more efficient at identifying legitimate transactions than fraudulent ones, which renders it ineffective when identifying new fraud cases.
Tradeoff: Recall vs. Precision
Our objective is to maximize recall and trade a bit of the precision, as it is less financially damaging to predict “fraud” on non-fraudulent transactions than to miss any fraudulent ones.
Solution:
Balancing the classes by resampling the minority upscale (fraudulent transactions) to have the same frequency as the majority class (non-fraudulent).
Classification Models
The dataset was split into train and test, in order to train our models and objectively measure their performance.
A series of diverse algorithms were computed to classify whether a transaction was deemed fraudulent or legitimate.
Models run were Logistic Regression, Random Forest, LGBM Classifier, Multi-layer perceptron (MLP), XGB, KNN, SVM & ADABoost.
The LGBM classifier excels in classification tasks, with high accuracy on both training and test sets. To improve performance, we’re using hyperparameter tuning. This technique fine-tunes the model to reduce overfitting and underfitting.
Using randomized search, we found the optimal parameters for our LGBM classifier, resulting in our accuracy increasing from 98.6% to 99.03%
Feature Importance
In this study, we aimed to understand the importance of each feature in determining fraudulent transactions using the best model we developed.
To achieve this, we ran a feature importance visualization, which allowed us to gain insight into the relative importance of each feature in the model.
The results of the visualization revealed that the two features emerged as the most significant attributes in determining fraudulent transactions are:
(1) “Time Diff between first and last (Mins)”: Time difference between the first and last transaction.
(2) “Unique received from addresses”: Total Unique addresses from which account received transactions.
(1) “Time Diff between first and last (Mins)”
“Time Diff between first and last (Mins)” can be a good indication of fraud on the blockchain because it can help detect suspicious activities that occur within a short period of time. For example, if a large number of transactions are made within a very short time frame, it could indicate that the transactions are being made by a bot or an automated script rather than by a human.
Additionally, it can be a sign of a coordinated attack where multiple transactions are made simultaneously to flood the network with fake transactions.
(2) “Unique received from addresses”
“Unique received from addresses” can be a good indication of fraud on the blockchain because it can help detect suspicious activities that involve multiple addresses.
For example, if a single transaction is made from many different addresses, it could indicate that the transactions are being made by someone who is attempting to evade detection. It could also indicate a case of a group of individuals working together to commit fraud, or a possible money laundering operation.
Moreover, having multiple sources of funding in a transaction, or many different “from addresses” could also be a sign of a transaction that was made by an entity that may not have the proper authorization to make the transaction, or an entity attempting to anonymize its identity.
These findings can assist organizations in allocating resources towards the detection of these specific attributes during the transaction monitoring process, ultimately leading to more efficient and effective fraud detection.
Furthermore, such visualization of feature importance can be useful for other researchers and practitioners in the field of fraud detection, providing a valuable starting point for further research and development.
https://www.linkedin.com/in/nmukhtar/
GitHub Code
Dataset